Dynamics of the Spin-Boson Model¶
Overview¶
In this notebook we will simulate the dynamics of a 1-mode spin-boson model.
We will first start with implementing it from scratch, and then show how to use the TimeEvolution
class for the very same task.
Determine the model and basis¶
TenCirChem provides a simple yet versatile interface to define the Hamiltonian and the basis set. The Hamiltonian operators are specified through a list of Op
object and simple harmonic oscillator (SHO) basis set is used for the boson in the spin-boson model. The relevant classes are borrowed from Renormalizer.
[1]:
from tencirchem import Op, BasisHalfSpin, BasisSHO
epsilon = 0
delta = 1
omega = 1
g = 0.5
ham_terms = [
Op("sigma_z", "spin", epsilon),
Op("sigma_x", "spin", delta),
Op(r"b^\dagger b", "boson", omega),
Op("sigma_z", "spin", g) * Op(r"b^\dagger+b", "boson"),
]
basis = [BasisHalfSpin("spin"), BasisSHO("boson", omega=omega, nbas=8)]
ham_terms, basis
[1]:
([Op('sigma_z', ['spin'], 0.0),
Op('sigma_x', ['spin'], 1.0),
Op('b^\\dagger b', ['boson', 'boson'], 1.0),
Op('sigma_z b^\\dagger+b', ['spin', 'boson'], 0.5)],
[BasisHalfSpin(dof: spin, nbas: 2),
BasisSHO(dof: boson, x0: 0.0, omega: 1, nbas: 8)])
tencirchem.dynamic.model
offers several shotcuts for common models
Quantum circuit is not able to simulate the boson basis, and proper basis transform to qubit basis is required
[2]:
# transform the Hamiltonian and basis
from tencirchem.dynamic import qubit_encode_op, qubit_encode_basis
boson_encoding = "gray"
ham_terms_spin, constant = qubit_encode_op(ham_terms, basis, boson_encoding)
basis_spin = qubit_encode_basis(basis, boson_encoding)
# boson basis is transformed to spin basis
ham_terms_spin, constant, basis_spin
[2]:
([Op('X', ['spin'], 1.0),
Op('Z', [('boson', 'TCCQUBIT-0')], -2.0),
Op('Z Z', [('boson', 'TCCQUBIT-0'), ('boson', 'TCCQUBIT-1')], -1.0),
Op('Z Z Z', [('boson', 'TCCQUBIT-0'), ('boson', 'TCCQUBIT-1'), ('boson', 'TCCQUBIT-2')], -0.5),
Op('Z X', ['spin', ('boson', 'TCCQUBIT-2')], 0.9517337620166572),
Op('Z Z X', ['spin', ('boson', 'TCCQUBIT-1'), ('boson', 'TCCQUBIT-2')], -0.040295934250509535),
Op('Z Z X', ['spin', ('boson', 'TCCQUBIT-0'), ('boson', 'TCCQUBIT-2')], -0.26872106012443797),
Op('Z Z Z X', ['spin', ('boson', 'TCCQUBIT-0'), ('boson', 'TCCQUBIT-1'), ('boson', 'TCCQUBIT-2')], -0.14271676764170976),
Op('Z X', ['spin', ('boson', 'TCCQUBIT-1')], 0.4829629131445341),
Op('Z X Z', ['spin', ('boson', 'TCCQUBIT-1'), ('boson', 'TCCQUBIT-2')], -0.4829629131445341),
Op('Z Z X', ['spin', ('boson', 'TCCQUBIT-0'), ('boson', 'TCCQUBIT-1')], -0.12940952255126037),
Op('Z Z X Z', ['spin', ('boson', 'TCCQUBIT-0'), ('boson', 'TCCQUBIT-1'), ('boson', 'TCCQUBIT-2')], 0.12940952255126037),
Op('Z X', ['spin', ('boson', 'TCCQUBIT-0')], 0.25),
Op('Z X Z', ['spin', ('boson', 'TCCQUBIT-0'), ('boson', 'TCCQUBIT-2')], 0.25),
Op('Z X Z', ['spin', ('boson', 'TCCQUBIT-0'), ('boson', 'TCCQUBIT-1')], -0.25),
Op('Z X Z Z', ['spin', ('boson', 'TCCQUBIT-0'), ('boson', 'TCCQUBIT-1'), ('boson', 'TCCQUBIT-2')], -0.25)],
3.5,
[BasisHalfSpin(dof: spin, nbas: 2),
BasisHalfSpin(dof: ('boson', 'TCCQUBIT-0'), nbas: 2),
BasisHalfSpin(dof: ('boson', 'TCCQUBIT-1'), nbas: 2),
BasisHalfSpin(dof: ('boson', 'TCCQUBIT-2'), nbas: 2)])
Construct ansatz¶
TenCirChem uses the variational Hamiltonian ansatz. Suppose the Hamiltonian has \(N\) terms and is written as
The the variational Hamiltonian ansatz has the form
where \(| \phi \rangle\) is the initial state, \(\theta_{kj}\) is the circuit parameter and \(M\) is number of layers for the ansatz.
[3]:
import tensorcircuit as tc
from tencirchem import set_backend
from tencirchem.dynamic import get_ansatz, get_jacobian_func, get_deriv
# dynamics simulation requires auto-differentiation from JAX.
set_backend("jax")
# the initial state
init_circuit = tc.Circuit(len(basis_spin))
# number of layers
n_layers = 3
# get the ansatz. Note that the spin basis is feed in
ansatz = get_ansatz(ham_terms_spin, basis_spin, n_layers, init_circuit)
# ansatz accepts parameters and outputs wavefunction
import numpy as np
ansatz(np.zeros(n_layers * len(ham_terms_spin)))
[3]:
DeviceArray([1.+0.j, 0.+0.j, 0.+0.j, 0.+0.j, 0.+0.j, 0.+0.j, 0.+0.j,
0.+0.j, 0.+0.j, 0.+0.j, 0.+0.j, 0.+0.j, 0.+0.j, 0.+0.j,
0.+0.j, 0.+0.j], dtype=complex128)
The jacobian matrix for the wavefunction \(\partial | \psi \rangle / \partial \theta_{kj}\) is required to perform VQA dynamics based on TDVP.
[4]:
# the funcction to evaluate the jacobian of the wavefunction
jacobian_func = get_jacobian_func(ansatz)
Compute time derivative \(\dot \theta\) based on McLachlan’s variational principle
Here \(M\) is a matrix and \(V\) is a vector
[5]:
# the Hamiltonian in dense matrix format
from tencirchem import get_dense_operator
h = get_dense_operator(basis_spin, ham_terms_spin)
# time derivative for $\theta_{kj}$ in the scipy solve_ivp format
def scipy_deriv(t, _theta):
return get_deriv(ansatz, jacobian_func, _theta, h)
Run simulation¶
Use SciPy RK45 solver to solve the \(\theta\) initial value problem (IVP). Calculate \(\langle \hat \sigma_z \rangle\) for plotting.
[6]:
from scipy.integrate import solve_ivp
from scipy.linalg import expm
# time step
tau = 0.1
# initial value
theta = np.zeros(n_layers * len(ham_terms_spin))
# operator to measure Z
z_op = get_dense_operator(basis_spin, Op("Z", "spin"))
z_list = [1]
# for reference
z_exact_list = [1]
for n in range(100):
# time evolution
scipy_sol = solve_ivp(scipy_deriv, [n * tau, (n + 1) * tau], theta)
# time evolved parameter
theta = scipy_sol.y[:, -1]
# calculate expectation
state = ansatz(theta)
z_list.append((state.conj().T @ z_op @ state).real)
state_exact = expm(-1j * (n + 1) * tau * h) @ init_circuit.state()
z_exact_list.append((state_exact.conj().T @ z_op @ state_exact).real)
Plot¶
[7]:
from matplotlib import pyplot as plt
import mpl_config
t = np.arange(101) * tau
plt.plot(t, z_list, label="Variational")
plt.plot(t, z_exact_list, linestyle="--", label="Exact")
plt.xlim(0, 10)
plt.ylim(-1, 1)
plt.xlabel("$t$")
plt.ylabel(r"$\langle \sigma_z \rangle$")
plt.legend(loc=(1, 0.75))
[7]:
<matplotlib.legend.Legend at 0x7ff5484ae950>
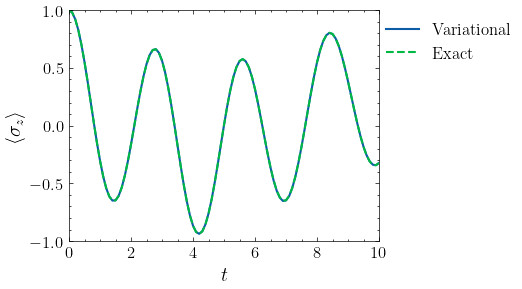
Use TimeEvolution
class¶
In this subsection we show how to use the TimeEvolution
class to greatly simplify the workflow.
[8]:
from tencirchem import TimeEvolution
te = TimeEvolution(ham_terms_spin, basis_spin, property_op_dict={"Z": Op("Z", "spin")})
for i in range(100):
te.kernel(tau)
[9]:
plt.plot(te.t_list, te.properties["Z"][:, 0].real, label="Variational")
plt.plot(te.t_list, te.properties["Z"][:, 1].real, linestyle="--", label="Exact")
plt.xlim(0, 10)
plt.ylim(-1, 1)
plt.xlabel("$t$")
plt.ylabel(r"$\langle \sigma_z \rangle$")
plt.legend(loc=(1, 0.75))
plt.savefig("sbm.pdf")
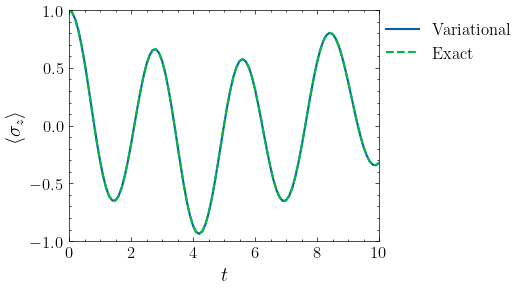